Towards Data Driven Design of Flow Blurring Atomizer
Liquid atomization relies on a variety of forces that disturb the surface of the liquid. In the case of flow-blurring (FB) atomization, turbulent structures are induced within the liquid channel to achieve this effect. It is known that the transition from the conventional flow-focusing (FF) pattern to the flow-blurring regime occurs at a specific geometric configuration and gas-to-liquid ratio (GLR). This transition causes the gas phase to flow partially upstream into the liquid channel, creating a back-flow mixing region. The behavior of internal recirculation is characterized by the gas penetration depth, GLR, and the geometry of the system. However, the dynamic nature of the turbulent structures responsible for generating transient micro-scale ligaments is still unclear, particularly in regards to the evolution of three-dimensional (3D) gas phase structures at the interface and their correlation with the shear acting on the liquid surface. To address this knowledge gap, the current investigation aims to establish a database of the transient nature of gas phase coherent structures across a range of operating conditions and nozzle designs. The primary objective is to shed light on the momentum transfer mechanism that underpins this process.
For that purpose, a 3D computational domain for the FB nozzle geometry is prepared, and alternative modelling strategies for gas phase turbulence in the COMSOL Multiphysics® environment were tested on the high-performance computer system, BW UniCluster 2.0. Preliminary analysis was followed by a second grid refinement study for the selected large eddy simulation (LES) model configuration. In the next phase of the work, the gas phase flow at different phases of the cyclic FB atomization process was modelled, considering various operating points and geometric configurations. Flow asymmetry and the 3D nature of the flow structures were assessed using similarity-based methods and recurrence plots. The transient shear distribution on the liquid surface and its dependence on flow regime shifts and geometry were quantified. By placing virtual pressure sensors in the annular region during the simulations, different machine learning (ML) models, such as Lasso, Support Vector Machines (SVM), Random Forests, and Artificial Neural Networks, were trained and tested. The aim was to explore the optimal sensor configuration for capturing informative signals and determine whether it is possible to estimate surface shear using simple pressure measurements in a real setup. The analysis revealed that it is not only possible to characterize the flow regime shifts with a single pressure sensor but also estimate the shear acting on the surface. Moreover, the use of multi-physics software like COMSOL® enables the creation of a digital twin of the complex process, which can be leveraged for designing a test rig.
Overall, this study contributes to a better understanding of the intricate dynamics involved in flow-blurring atomization. The outcomes have implications for optimizing atomizers in various applications, such as combustion systems and spray coating processes. Additionally, the integration of machine learning techniques and simulation tools opens up possibilities for improving the efficiency of atomization simulations and guiding future experimental investigations.
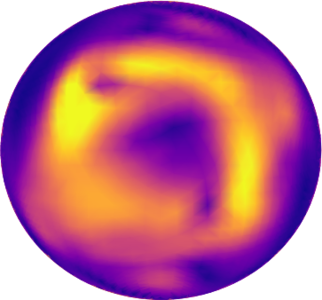
Download
- Ates_et_al_COMSOL_2023.pdf - 0.73MB
- Ates--COMSOL-Conference-2023-munich.pdf - 1.03MB